Video analytics is a technology that processes a digital video signal using a special algorithm to perform security and associated functions. It automatically analyzes videos to detect and determine temporal and spatial events. By leveraging existing video surveillance infrastructure, video analytics is a highly cost-effective means to augment security with new detection capabilities, reduce staffing requirements by directing attention to key events, automate access control and other facility functions, and collect customer behavior-related data.
Video analytics is extensively used across several end-use sectors, including banking, financial services & investment, education, aerospace & defense, healthcare, manufacturing, retail, automotive, transportation & logistics, government, agriculture, and other sectors such as event management, hospitality, and entertainment. The features offered by video analytics, such as automated surveillance, crime detection & prevention, access control, face recognition, traffic monitoring, and easier crowd management, significantly encourage the use of video analytics systems across the end users. Furthermore, as an impact of the COVID-19 pandemic, the market experienced a high adoption of video analytics for crowd management, social distancing, face mask detection, and other similar purposes.
Video analytics solutions proved crucial in containing the spread of the coronavirus. Civil authorities used video surveillance cameras to monitor public places and identify people without masks. The solutions were also used to monitor airports, metro stations, malls, and other crowded places such as parks, schools, and universities. Moreover, governments also adopted facial recognition software to detect people with and without masks. Video analytics solutions helped civil authorities by enabling quick detection & identification of people, improving their overall efficiency and public safety. Government authorities also used video analytics technology to manage crowds.
Advancements in deep learning technology have significantly improved the efficiency of video surveillance and analytics. Deep learning technology has improved the accuracy of face recognition. According to the National Institute of Standards and Technology (NIST), as of April 2020, the best face identification algorithm had an error rate of just 0.08% compared to 4.1% in 2014. In ideal conditions, facial recognition systems can have near-perfect accuracy. Verification algorithms used to match subjects to clear reference images (such as a passport photo or mugshot) can achieve accuracy scores as high as 99.97% on standard assessments like NIST’s Facial Recognition Vendor Test (FRVT). These results are comparable to the best results of iris scanners. Thus, face verification has become so reliable that even banks feel comfortable relying on it to log users into their accounts. In addition, person detection & object detection are other areas where deep learning has shown tremendous progress. Therefore, the technology is widely implemented at airports, metro stations, and other public areas to detect and track people with criminal records. Increased accuracy & reduced false alarms have also boosted the adoption of video analytics.
Furthermore, deploying edge computing devices powered by AI-optimized surveillance hard drives has several benefits, such as reductions in data access delays, minimal bandwidth issues, and efficient data compliance handling. Such edge devices powered by AI-enabled drives deliver faster insights on-site, enabling critical, real-time decision-making. Thus, video analytics solutions are useful in traffic management, airport security, and other real-time surveillance applications.
The video analytics market has witnessed the consistent emergence of technology trends in recent years. Various industries are combining other technologies with video analytics to improve overall business performance. Location (spatial) analytics is one such technology that, when combined with video analytics, improves the efficiency of video analytics considerably.
Following are the key trends observed in the global video analytics market:
There are some situations where a camera cannot act due to visual obstacles that are not included in camera tampering algorithms, meaning that video analytics will not work. The situation can be beyond the line of sight. In such situations, combining video analytics with other advanced technologies, such as real-time location systems (RTLS) or radio-frequency identification systems (RFID), can provide the exact data or location. This helps fill the gap between the actual video footage and the video data required for the analysis. Microsoft’s Azure Cognitive Service offers the spatial analysis capabilities of computer vision, which can be used in conjunction with live video analytics on IoT edge to better understand the spatial relationships between people and movement in physical environments. This allows the user to count people in a designated zone within the camera’s field of view, track a person crossing a designated line or area, or when people violate a distance rule. The live video analytics module captures live video from real-time streaming protocol (RTSP) cameras and engages the spatial analysis module for AI processing. These modules can be configured to enable video analysis and clip recording locally or on Azure Blob storage.
The retail sector is at the forefront of adopting video analytics. Retail businesses adopt video analytics to improve sales and in-store customer experience. Using video analytics, enterprises in the retail industry can understand consumer behavior, attitudes, and preferences. Consumers’ buying behaviors change frequently, and merchants must adapt and innovate as per new trends and customer preferences to stay competitive. Furthermore, retailers can optimize product placement and customer traffic flow to improve sales by understanding how customers typically navigate through the in-store space and how long they spend at certain locations. Data-driven video business intelligence enables merchants to quickly see and distill down patterns of in-store behavior so that they can design a more efficient store layout.
New business intelligence derived through video content analysis can also be used to formulate effective promotions and display designs that facilitate stronger engagement. By identifying trends in engagement with product displays, merchants can better correlate promotions, displays, and sales. Additionally, managing store traffic by leveraging video analytics to understand in-store traffic patterns & peaks, streamlining checkout at all points using video content analysis to eliminate conversion barriers by preventing long lines from forming, and using machine learning technology, video content analytics solutions help quantify and classify individuals shopping in stores.
Download Free Research PDF @ https://www.meticulousresearch.com/download-sample-report/cp_id=5274
Related Tag:
Related Blogs:
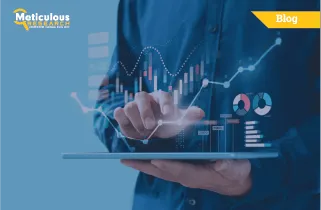
Growing Need for Cybersecurity Services Among SME's
Read More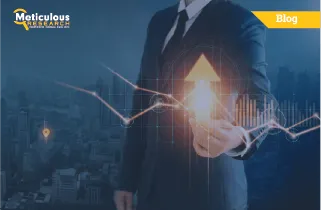
Benefits of Cloud-Based Industrial Asset Management Solutions
Read More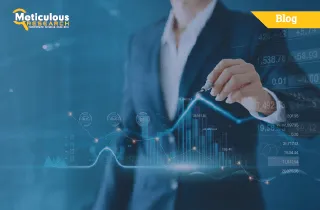
Increasing Importance of English Language to Drive Market Growth
Read More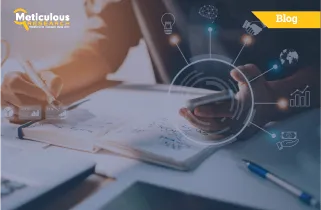
Video Analytics for Enhanced Surveillance
Read More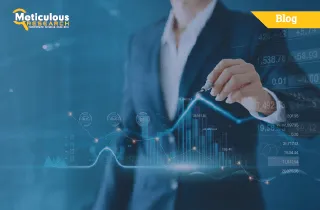
Mobile Computers for Efficient Data Collection
Read More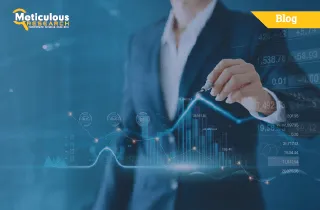
AI-based Cybersecurity Solutions in the BFSI Sector
Read More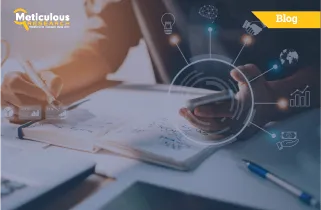
RFID Technology among Manufacturing Industries
Read More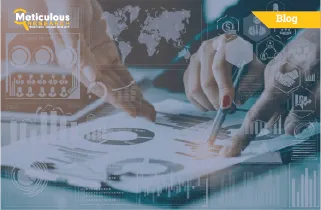
Development of Smart Infrastructure
Read More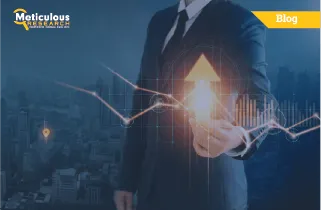
Growing Security Requirements in the BFSI Sector
Read More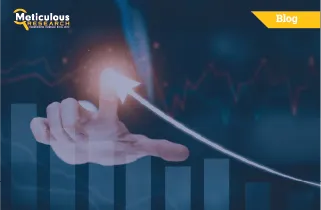
Rising Need for Home Safety & Security
Read More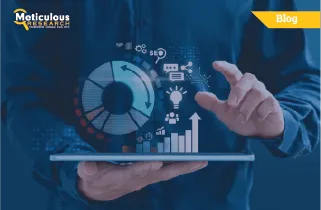
Zero Trust Networking
Read More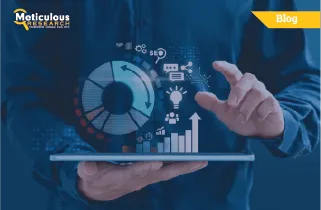
Factors Affecting Speech and Voice Recognition Market Growth
Read More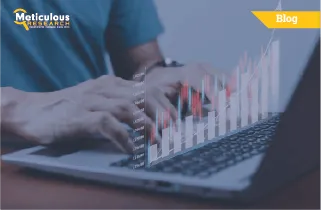
Growing Adoption of Cloud Infrastructure in the BFSI Sector
Read More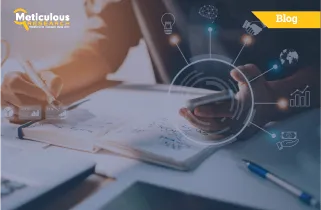
Data Protection Regulations Boosting the Adoption of Digital Vaults
Read More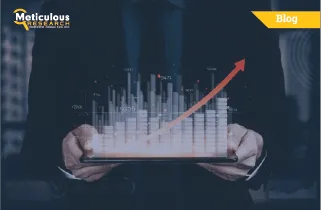
Growing Demand for Chemical Surface Treatment Solutions
Read More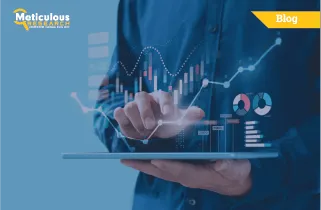
Growing Need for Cybersecurity Services Among SME's
Read More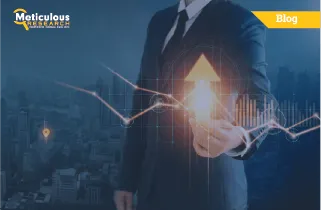
Benefits of Cloud-Based Industrial Asset Management Solutions
Read More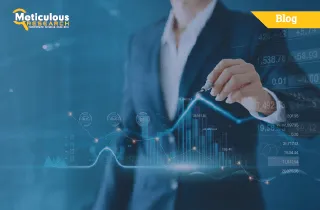
Increasing Importance of English Language to Drive Market Growth
Read More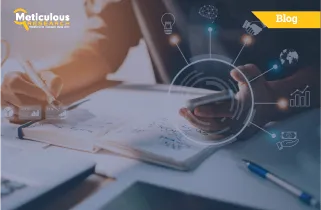
Video Analytics for Enhanced Surveillance
Read More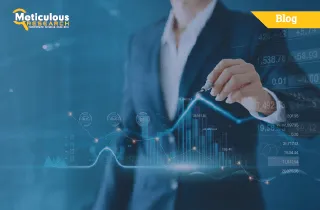
Mobile Computers for Efficient Data Collection
Read More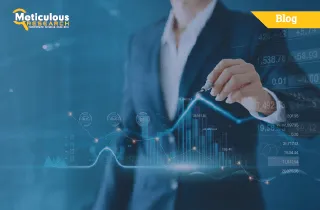
AI-based Cybersecurity Solutions in the BFSI Sector
Read More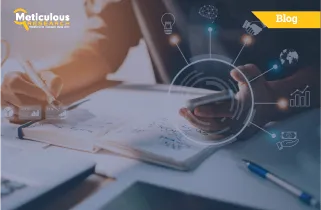
RFID Technology among Manufacturing Industries
Read More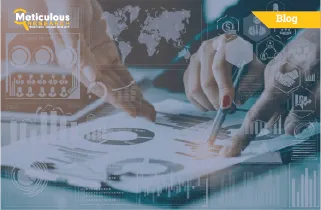
Development of Smart Infrastructure
Read More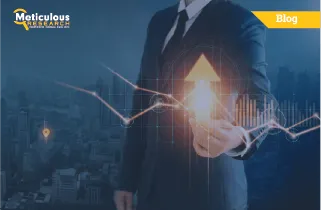
Growing Security Requirements in the BFSI Sector
Read More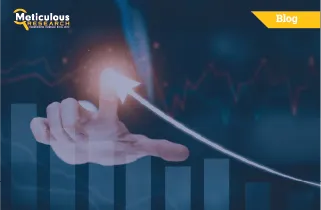
Rising Need for Home Safety & Security
Read More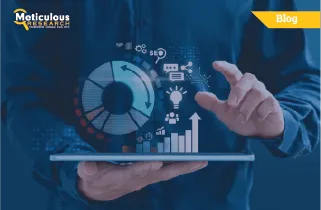
Zero Trust Networking
Read More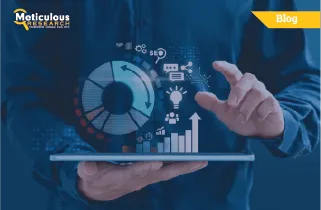
Factors Affecting Speech and Voice Recognition Market Growth
Read More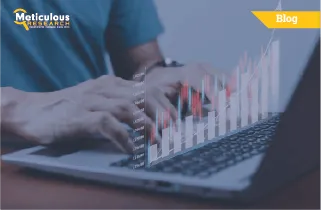
Growing Adoption of Cloud Infrastructure in the BFSI Sector
Read More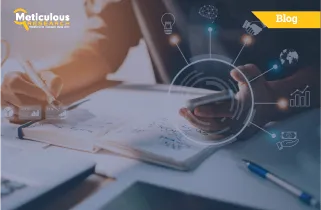
Data Protection Regulations Boosting the Adoption of Digital Vaults
Read More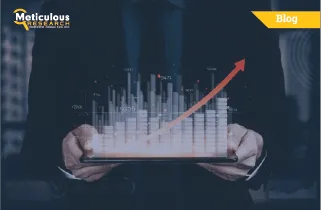
Growing Demand for Chemical Surface Treatment Solutions
Read More